Why Specialization is Essential for Data Scientist: Unlocking the Key Benefits
September 12, 2024 2024-09-12 10:27Why Specialization is Essential for Data Scientist: Unlocking the Key Benefits
With the rise of data-driven decision-making in businesses across all sectors, data science has become a critical component of organizational strategy. The field has evolved significantly from its early days as a broad, generic field. Data science encompasses a wide range of specialized areas, each designed to address distinct challenges and problems. While having a broad understanding of data science is beneficial, specializing in a specific domain can significantly enhance your career prospects. This article explores what constitutes a data science domain, why it is important, and the factors driving its growth. Specializing in a particular area of data science not only enhances your skill set but also opens up diverse career opportunities. We will also look into the various specializations within data science and how choosing the right one can impact your career as a data scientist.
Data science as a multidisciplinary field
Data science is a multidisciplinary field that blends machine learning engineering, data engineering, statistical analysis, and domain expertise to extract valuable business insights from both structured and unstructured data. It leverages variety of tools, algorithms, and machine learning techniques to uncover patterns and make data-driven decisions. As the field has expanded, it has become clear that mastering data science requires more than just a generic base; it demands specialized skills and domain specificity to address distinct problems effectively. Specialization allows you to build a reputation as a trusted expert, making it easier to showcase your competence and advance within your preferred field. It also provides you with the opportunity to work on projects that align with your interests and strengths, leading to greater job satisfaction and career fulfillment.
Why specialization is essential for any data scientist?
- Being a generalist data scientist has its advantages, possessing a broader understanding of various domains, technologies and methodologies. This enables them to connect ideas across disciplines and identify patterns that specialists might overlook. However, benefits of data science specialization offer a multitude of benefits. By honing skills in a specific area, data scientists can become trusted industry experts and develop a deep understanding of the intricacies and nuances within the specified domain. The specialization allows data scientists for more focused research, problem-solving, and innovative approaches, leading to impactful insights and solutions. Also, companies seek specialists to tackle complex challenges in their respective fields.
- Interdisciplinary collaborations
Furthermore, data science specialization promotes interdisciplinary associations. Data scientists from various specializations can come together to leverage each other’s expertise, promoting innovation and driving solutions that transcend the boundaries of their individual domains. As the field of data science continues to expand, we can expect to see more specialized professionals who not only provide in-depth insights but also actively contribute to the broader landscape of interdisciplinary data-driven advancements. In essence, data science specialization is not just a trend; it is a fundamental aspect of the evolution and maturation of the data science field.
- Requirement evaluation
Data scientists with specialization knowledge can identify business needs and corresponding solutions more effectively. They understand the business problem-specific requirements and can tailor their data science techniques accordingly. This leads to better insights and more accurate decision-making.
- Reduction in the training time
Hiring data scientists with specialization expertise can significantly reduce training time and costs for organizations. When professionals already have industry-specific knowledge, they require less training to understand the domain-specific challenges and processes. This increases the team’s efficiency and allows organizations to work on more projects with better outcomes in less time.
- Better earning potential
When you gain data science specialization, it positions you as an expert in your field, even before joining an organization. It gives you a competitive advantage and helps you command a higher salary and better job opportunities. With specialized knowledge, you can confidently present yourself as someone with industry-specific expertise, which is highly valued by employers.
Factors Driving Data Science Specialization
- Complex problem-solving needs – Specialized fields often present intricate and unique problems that require deep, domain-specific specialization to address effectively.
- Technological progress – Technological enhancements such as machine learning and artificial intelligence a demand for professionals adept at using these tools to tackle specific challenges
- Data proliferation – The rapid expansion of data across industries necessitates experts who can manage and extract meaningful insights from specialized data sources.
- Business impact – Organizations are increasingly recognizing the strategic value of data science, driving the need for specialists who can optimize data-driven strategies and maximize the ROI.
- Regulatory and ethical demands – Different sectors face unique regulatory and ethical requirements, making it important to have specialist data scientists who can traverse these complex landscapes effectively.
Prominent areas of Data Science Specialization
Here are some of the specialized domains depicting how data science expertise is applied to solve distinct business problems and seize opportunities in various industries.
- Quantitative finance – Data scientists in finance use techniques like predictive modeling, risk assessment, and algorithmic trading to guide investment decisions, detect fraud, and enhance financial processes.
- Healthcare and medical data science – This specialization involves analyzing extensive patient data to improve healthcare outcomes, streamline hospital operations, and advance medical research. Techniques such as predictive modeling and data-driven decision-making are essential.
- E-commerce and recommendation systems – Data scientists working in e-commerce develop personalization strategies, recommendation algorithms, and market analysis to enhance customer experience, enhance sales, and optimize supply chains.
- Social media analysis – This specialization involves extracting insights from social media data to understand user behavior, conduct sentiment analysis, and predict viral content. Applications include marketing strategies, brand management, and public opinion analysis.
- Transportation and logistics – Data scientists in this sector focus on optimizing routes, managing fleets, and improving supply chain efficiency to reduce costs, enhance delivery times, and minimize fuel consumption.
- Cybersecurity and threat detection – Data scientists in cybersecurity analyze network traffic and user behavior to identify and mitigate cyber threats. They use machine learning to detect patterns and anomalies indicative of security breaches.
Conclusion
The evolution of data science into specialized domains underlines the field’s maturity and its ability to adapt to the diverse needs of different industries. Specialization becomes a key driver of innovation, offering data science professionals the chance to make significant impacts in their respective fields.
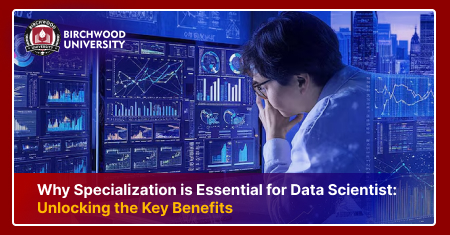