The Role of Text Mining and Sentiment Analysis in Shaping Marketing Strategies
Dec 13, 2024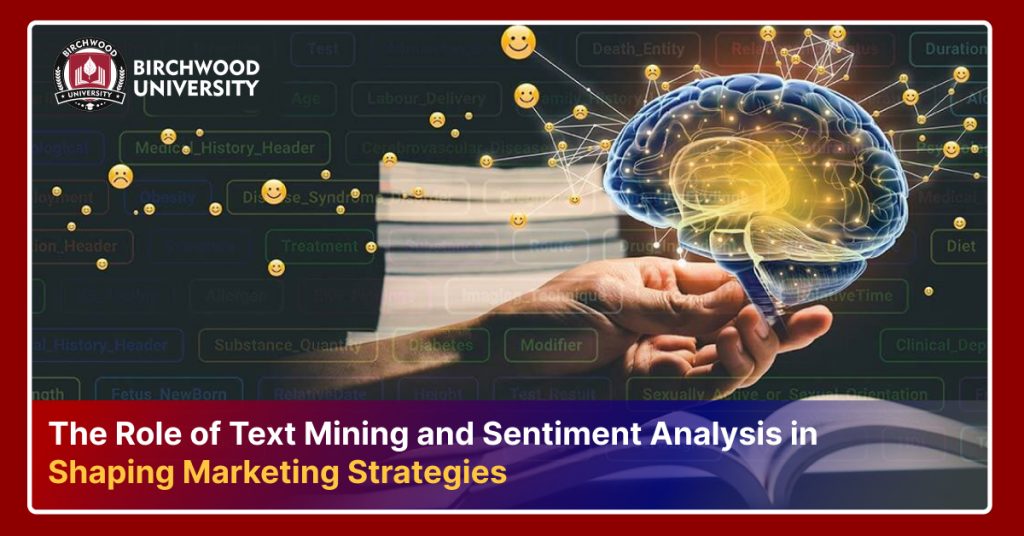
Businesses are usually flooded with huge amounts of data, and textual data is one of the most precious resources for marketers. Customer reviews, social media posts, emails, and blogs are full of unstructured text that provides crucial information regarding customer behavior, preferences, and sentiments. To utilize this potential, businesses make use of two powerful techniques: text mining and sentiment analysis. The approach not only shows better insights into the attitudes of the consumer but also helps companies develop effective, data-driven marketing strategies. This article discusses how text mining and sentiment analysis determine marketing strategy and how one can exploit these techniques to succeed in business.
Text mining for unlocking insights from unstructured data
Text mining is the retrieval of valuable information and patterns from unstructured text. Unlike conventional data analytics which focuses mainly on structured data such as numbers and categories, text mining involves qualitative data like customer feedback, posts on social media, emails, and reviews on products, among others. The said unstructured text is made actionable using NLP algorithms and this actionable information supports a better-informed decision or strategy.
Steps in performing text mining for marketing strategies
1. Data collection: The process initiates with collecting data relevant for text mining. Marketing professionals collect text data from customers' opinions on any product, their reviews over the internet, various social networking sites, or through customer surveys. Social media listening tools like Brandwatch, Sprout Social, or Hootsuite enable tracking all online conversations regarding a brand or any product across social media websites.
2. Data pre-processing: After the text is collected, it needs to be cleaned and subsequently pre-processed. The process includes removing irrelevant information, correcting spelling mistakes, and removing stop words - the most frequent words in a language like "the", "and" "is". This makes the text ready for analysis.
3. Text analysis: Next, the text is analyzed using various techniques. Common methods include:
• Keyword extraction: Most frequently appearing terms to identify trends, concerns or interests.
• Topic modeling: Categorizing the text into topics to uncover underlying themes or patterns.
• Named entity recognition (NER): The identification of entities, such as brand names, products, locations, or people, contained within a text.
4. Data visualization: In general, mined results of text are presented using visualizations like word clouds, bar graphs, or heatmaps, making it easier to identify trends and patterns.
5. Actionable insights: They can be used to streamline business marketing strategies. For example, if a product review mentions "durability" and "quality" quite frequently, then a company can play upon these aspects in their subsequent marketing campaigns.
Examples of text mining in marketing strategies
1. Customer complaints and reviews: Companies may analyze reviews on Amazon or Yelp. Thus, a hotel chain may find that cleanliness and friendly staff are frequently mentioned and develop targeted marketing campaigns from these insights.
2. Brand perception on social media: There is enormous unstructured data in social media. Brands can mine posts, comments, and tweets to understand what customers feel about them. An apparel brand might analyze the Instagram post for trends such as "sustainable fashion", thus guiding content marketing and influencer partnerships.
3. Market segmentation: Text mining helps identify customer segments based on language patterns. For instance, a tech company might find that one segment values "battery life" while another focuses on "camera quality," allowing for customized marketing campaigns.
Sentiment Analysis - Understanding consumer emotions
Sentiment analysis falls under text mining as a subgroup that emphasizes finding the underlying emotional tone in a succession of words. It usually categorizes the text of a brand or service product as positive, negative, or neutral. Marketing people, thus, understand if customers are in favour or against it. By finding out customer opinions, they can come to know about what people say and with what kind of feelings.
Phases of implementing sentiment analysis for marketing strategies
1. Data collection: Similar to text mining, the initial step in sentiment analysis is collecting relevant text data. This can be sourced from online reviews, social media posts, blogs, and customer surveys.
2. Data pre-processing: The preprocessing involved in sentiment analysis is almost like text mining, where one removes unwanted information and makes the text ready for analysis.
3. Sentiment detection: It is the process through which NLP algorithms use to identify whether the given text is positive, negative, or neutral. It looks for sentiment-bearing words ("happy", "love", "disappointed", and "hate") and examines their context.
4. Classification: After analyzing the text, it is classified into categories of sentiments - positive, negative, or neutral. More advanced sentiment analysis can even identify specific emotions like joy, anger, sadness, or fear.
5. Analysis and action: With the sentiment data, marketers can measure how consumers are responding to marketing campaigns, products, or services. If a product receives negative sentiment consistently, the company can investigate the cause and make adjustments accordingly.
Instances of sentiment analysis in marketing strategies
1. Product launches: Sentiment analysis can help measure the responses of consumers towards newly launched products. With a new smartphone, for instance, a company can track social media or reviews to check whether reactions are positive or negative. If there is negative sentiment, then in subsequent releases, they can correct problems such as technical glitches.
2. Campaign effectiveness: Sentiment analysis is used to evaluate the campaign's effectiveness. If there's a positive sentiment, that means the campaign is successful, but a negative one suggests the need for a message or strategy change.
3. Customer service: The sentiment can be analyzed on customer service interactions. Negative sentiment in chat transcripts indicates the company needs to address the customers' gripes immediately to enhance the quality of service.
4. Brand monitoring: Real-time tracking of public perception is possible with the help of sentiment analysis. Thus, a food company may track tweets regarding its brand and respond quickly if there is an emergence of negative sentiment because of a recall.
Blending text mining and sentiment analysis for building insightful marketing strategies
The combination of text mining and sentiment analysis offers a powerful, data-driven approach to crafting marketing strategies. For instance, when a company launches a new smartwatch, it can use text mining to identify features customers mention most, like battery life or design. Then, sentiment analysis can reveal customer emotions tied to these features. If feedback shows positive sentiment toward the "battery life" but negative sentiment about the "price," the company can adjust its strategy. It could focus its marketing on exceptional battery life while offering discounts or promotions to address the pricing concerns. This enables businesses to track real-time sentiment shifts, identify emerging trends, and adjust strategies to stay relevant and responsive.
Conclusion
Text mining and sentiment analysis, as a part of machine learning techniques, are transforming the marketing strategies of organizations by extracting actionable information from unorganized data and understanding consumer sentiments. Companies are able to make better decisions, personalize experiences, and communicate better with these techniques. Analyzing feedback, social media, and campaigns enables companies to develop strategies that create a more meaningful customer relationship and give an edge over the competitors.