The Power of AI Inferencing: The Heartbeat of Smart Decisions
Dec 12, 2024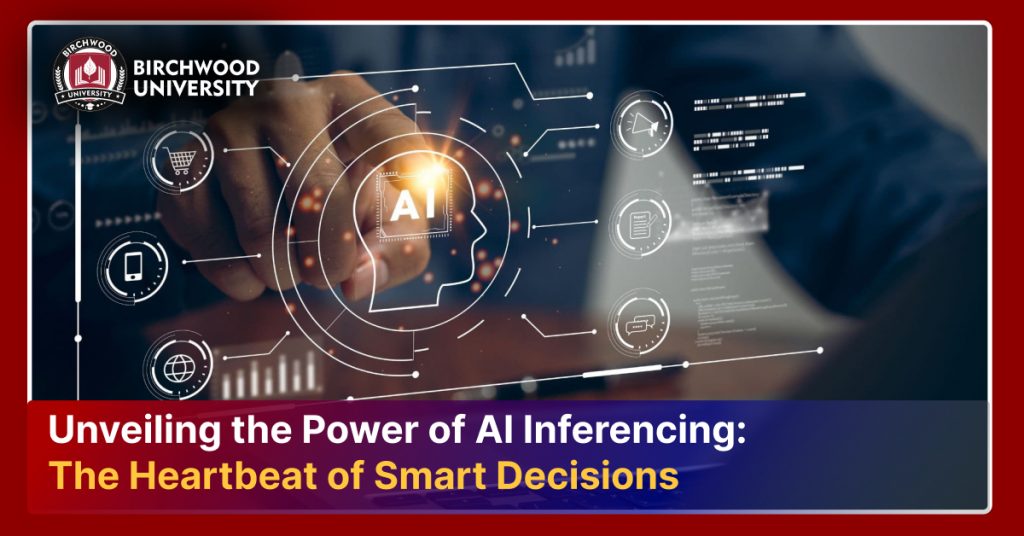
Artificial intelligence (AI) has emerged as one of the integral elements of modern technology. There is, however, a basic yet quintessential feature of artificial intelligence that often flies under the radar, known as AI Inferencing. While everyone from students to researchers, businessmen to technopreneurs has witnessed the capabilities of artificial intelligence in all aspects i.e. to predict, recommend, and automate actions or tasks, but truly transforming these actions into intelligent decision-building in real-time is done with AI Inferencing.
We shall dissect the magic behind AI Inferencing, introducing you to its essential parts and real-world applications in this article.
What is AI Inferencing? The secret behind AI’s real-time brainpower
AI Inferencing is a process of running a trained machine learning model against new, unseen data with the intent to make some predictions or decisions. That is basically the brain of AI where all the knowledge learned will be put to action. It occurs once the initial training phase is done with the model when the AI "learns" patterns from vast data. The model can thus infer or predict future patterns of data.
In simple terms, it is how AI takes all the knowledge gained from past experiences and gives an expression to them at present to make immediate, suitable and adapted decisions.
The mechanics of AI Inferencing: How does it work?
AI Inferencing is a process that includes several steps and components that work together to generate insights:
1. Training phase: This is where the process begins. AI models are trained using large datasets, allowing them to "learn" the underlying patterns and relationships in the data. For example, a facial recognition system might learn to identify faces based on thousands of photos.
2. Deployment of model: The model, once trained, is deployed into the production system that could be a smartphone app, an autonomous vehicle or financial monitoring tool. In this phase only the Inferencing takes place.
3. Inference phase: In the final stage, the learned model delivers decisions based on the data received in real-time by it, such as images, pictures, voice commands, and sensor data. For example, a self-driving car derives decision-making based on sensor readings from its surroundings to avoid accidents.
The inherent value in AI Inferencing is defined by the real-time decision-making or immediate feedback in action with many industries, such as healthcare, autonomous driving, and finance, requiring this capability for integration into their systems.
Prominent features of AI inferencing: Speed, accuracy, and scalability
The effectiveness of AI Inferencing is that it can make fast, accurate, and scalable predictions in real time. Here's why these features matter:
1. Speed: AI systems have to provide quick answers, as in real-time applications such as fraud detection and autonomous driving. Faster Inferencing means quicker decision-making.
2. Accuracy: Accuracy is paramount, though speed also counts. An AI system will have to infer whether a specific pattern in an MRI scan indicates a health risk accurately in healthcare diagnostics.
3. Scalability: Once trained, AI models can be deployed at scale, processing large volumes of data from different sources (millions of users on e-commerce platforms, for instance).
AI Inferencing at the Edge: Beyond the cloud
While traditional AI Inferencing occurs in the cloud, Edge Computing has brought Inferencing closer to the data source. Edge Inferencing occurs directly on local devices (smartphones, cameras, sensors), which allows for:
1. Reduced latency: Instantaneous decisions can be made without relying on a central server, which reduces the delay in decision-making.
2. Better privacy: As the data is processed on-site, sensitive information need not be transferred to a central server for processing, thereby improving the data's security and privacy.
3. Speed: Devices can decide instantaneously without requiring constant internet access.
For instance, in Google Pixel phone, on-device AI Inferencing is used for features like real-time language translation, object recognition, and even camera enhancements.
Diverse applications of AI Inferencing: Transforming industries
1. Autonomous vehicles: The self-driving car employs AI Inferencing to make real-time decisions based on sensor data from cameras, radar, and LiDAR. The system can analyze road conditions, pedestrian movements, and traffic patterns to make split-second decisions such as avoiding an obstacle or stopping at a red light. The key parameters required to be fast and accurate in Inferencing are critical to ensure safe driving.
2. Healthcare: AI Inferencing is changing the way of medical diagnostics. It is a model that's been trained on millions of images and can look at a patient's X-ray or MRI to find lung cancer or eye disease, for example. It accelerates diagnosis and gives greater accuracy, which can be very useful in saving lives as early as possible.
3. E-Commerce: Amazon and Netflix use AI Inferencing to give personalized recommendations. With a user's past behavior, AI infers what products or content they may prefer next and delivers it in real-time. This dynamic adjustment increases customer satisfaction and engagement.
4. Finance: Artificial Intelligence Inferencing detects fraud for finance by checking transactional data in real-time. The system marks suspicious activities as the AI model comes to know the patterns learned from the behavior of a user. If the activity goes against the usual, then the AI model can immediately trigger an alert or block a transaction and prevent fraudulent activities.
5. Smart assistants: Siri, Alexa, and Google Assistant rely on AI Inferencing to interpret user commands in real-time, thus providing accurate responses and the ability to complete tasks, enhancing convenience and user experience in daily activities.
Challenges in AI Inferencing: Overcoming the hurdles
AI Inferencing also presents key challenges.
- Data privacy concerns: Real-time Inferencing would require access to sensitive data, which raises privacy and security issues.
- Bias: The occurrence of wrong or discriminatory decisions based on biased or insufficiently trained data.
- Computational power: Deep learning in real-time Inferencing requires huge computational resources and specialized hardware like GPUs or AI accelerators to process.
Conclusion
AI Inferencing is revolutionizing the real-time decision-making process, providing efficiency, personalization, and security. It is only going to define the future of AI industries as it develops, enabling unprecedented precision and speed for systems to make decisions. As this technology evolves, its applications across various sectors will continue to expand, enhancing automation, improving user experiences, and driving innovation, ultimately transforming how businesses and consumers interact with intelligent systems.