Exploring the Role of AI in Predictive Analytics for Business Growth
December 9, 2024 2024-12-09 10:59Exploring the Role of AI in Predictive Analytics for Business Growth
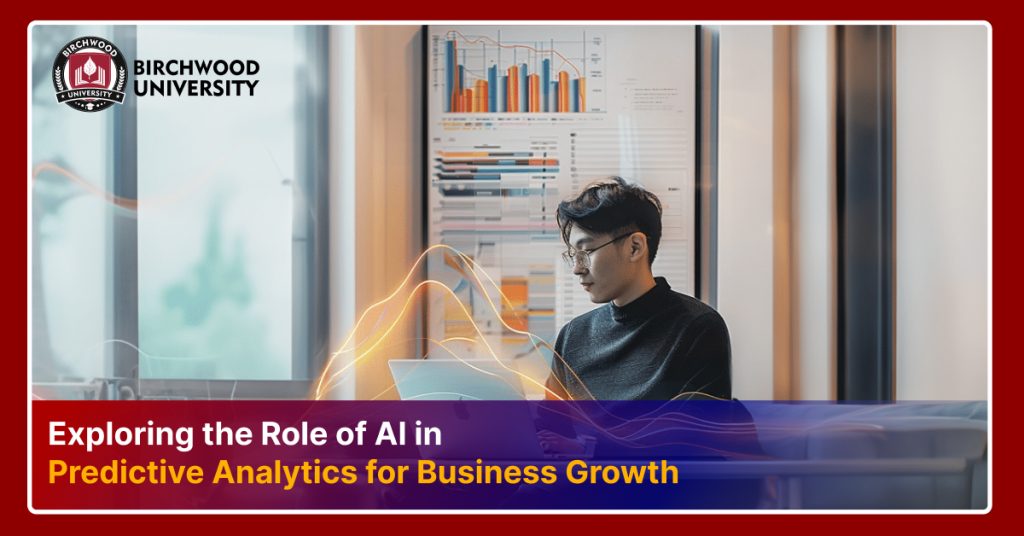
Business enterprises today have an enormous wealth of data about their operations and customers. From transaction information to manufacturing and shipping to customer, every activity leaves a trail of information that can be converted into valuable insights when accurately and efficiently leveraged. The intelligent use of this information can fuel business expansion, allowing prediction of future trends. Predictive analytics has become a key strategy for harnessing the treasure trove of data to improve business operations. From enabling businesses to manage customer relationships to optimizing business efficiency, and identification of dealing with business issues, predictive analytics significantly helps business thrive and sustain growth in a rapidly evolving landscape.
The arrival of AI has revolutionized predictive analytics, enabling businesses to know when to replenish their inventory, identify hidden patterns, predict customer churn, and draw highly accurate predictions. Business are endowed with these advanced, cutting-edge advantages with the integration of AI in predictive analytics. Through this blog, let us explore the role of AI in predictive analytics and learn how businesses are empowered today and are staying ahead of the competition.
Understanding Predictive Analytics and AI Integration
Predictive Analytics is the process of analyzing historical and real-time transactional data through the collective use of statistical algorithms, predictive modelling, and data mining, to draw predictions about future outcomes. It enables business to get an in-depth evaluation and understanding of their customer behavior and make necessary informed decisions, based on the insights. The inclusion of AI in the process of predictive analysis has enabled businesses to scan through vast amounts of data accurately and accelerate the process altogether.
Core components of AI in Predictive Analytics
Data: Data is key for training AI models. The data are collected from various sources including websites, sales records, customers interaction on the website and other social media platforms.
Algorithms: To evaluate data and make predictions
Predictions: Outcome of the AI algorithm that forecast future trends.
Roles of AI in Predictive Analytics
AI in predictive analytics imply the application of artificial intelligent techniques in the analysis of the historical data. AI methodologies and techniques are employed in extracting insights and detecting patterns from the colossal amounts of data. Let’s take a look at the roles of AI in predictive analytics and learn how AI powers the process.
Data Processing: Predictive analytics requires accumulation of a large amount of relevant data from diverse sources, from social media platforms to sensors to transactional records. AI deploys its algorithm in cleaning, normalizing and structuring the data to make it suitable for analysis.
Selection of Feature and Engineering: Another significant role of AI in predictive analytics is its function of identifying and constructing the most relevant variables (features) from the data which indicate future outcomes. The machine learning techniques of AI, like principal component analysis or decision trees, are deployed in this step to establish the most predictive attributes.
Deep Learning Techniques: AI enables predictive analysis of complex data sets by deploying deep learning models like layered neural networks. Deep learning models enables the capturing of intricate patterns and relationships from complex datasets like images or sequential data, which otherwise may be missed in the traditional simpler model.
Machine Learning Models: Predictive analytics with AI are powered by Machine learning models. Some of the most common models include linear and logistic regressions which are for continuous outcomes and binary outcomes respectively, and classification algorithms. These models can be supervised as the model learns from labeled data. Clustering, which is an unsupervised model, is deployed to detect hidden patterns in data without priorly-labelled outcomes.
NLP for Textual Analysis: AI techniques like Natural Language Processing are critical for analyzing data that are textual. The tool is used for understanding human language. Businesses with customer-intensive services need NLP for sentiment analysis and topic modelling, to mention a few. Analytical tasks that involve and requires understanding of textual data deploys NLP.
Time Series Analysis: For predictive analytics that needs to make forecast or future values in times series largely uses AI models like Long Short-Term Memory (LSTM) or AutoRegressive Integrated Moving Average (ARIMA). Time-dependent sequences like Weather data, stock prices, etc.
Continuous Learning and Adaptation: AI models allows improvement and efficiency in predictive analytics as it has the scope for feedback loops. It allows models to learn continuously and adapt from new data, thereby improving their relevance and accuracy overtime.
Benefits of Integrating AI in Predictive Analytics
AI-powered predictive analytics helps business gain massive growth and expansion. The following benefits enable businesses to achieve significant improvement across its operations.
High Customer Retention: Businesses that rely on AI-driven predictive analytics experience increased rates of customer retention. They are better informed about their customer behavior, patterns and preferences. With these insights, businesses can personalize interaction and tailor services as per their preferences and needs, and establish stronger relationships, leading to more confidence and loyalty from the customers.
Personalized Experience for Customers: AI-driven analytics provide insights of individual customer preferences, enabling businesses to deliver customized products, services and marketing campaign as well as interaction in the customer support.
Use cases of AI-driven Predictive Analytics in Business
Cost Savings
- Cutting down unnecessary expenses enabled by accurate forecasting
- Optimizing resource utilization
- Streamlining business processes
- Reducing wastes
Informed-Decision Making
- Informed decision powered by hard facts/data
- More accurate predictions
- Better resource allocation
- Enhanced outcomes
Inventory Management
- Advising on optimal stock levels
- Predicting future sales
Optimized Customer Service
- Insights to customer preference
- Personalized recommendation and targeted campaigns
Supply Chain Management
- Supply chain disruptions prediction
- Optimizing logistics and Inventory
Dynamic Pricing
- Adjusting prices in real-time
- Maximizing profits
- Optimizing market competitiveness
Sentiment Analysis and Management of brand reputation
- Real-time monitoring of customer online reviews and conversations
- Insights into public perception
- Prompt addressing of customer issues and queries
Conclusion
With business landscape becoming globalized and increasingly reliant on technology, AI-driven predictive analytics has become essential to sustain and thrive. From allowing business enterprises to identify unique talents to making informed decisions about their operations, the marriage of AI and predictive analytics is poised to transform businesses in ways never imagined. Businesses are equipped to unlock new growth opportunities and gain a competitive edge.
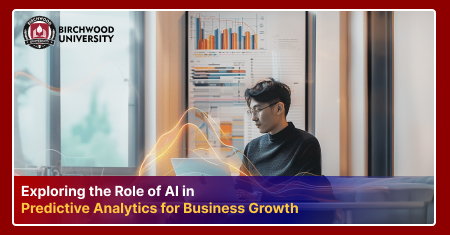