The Ultimate Guide to Domain-Specific AI
November 27, 2024 2024-11-27 6:38The Ultimate Guide to Domain-Specific AI
Businesses across domains are drowning in data but struggling majorly to extract real value from it. Managers handling business teams are under constant pressure to make smarter decisions that are quick and effective, manage various business risks, and gain a competitive advantage. Yet, despite having access to more information than ever before, many leaders are perplexed by noise in the data and unable to discern what truly matters.
This is where domain-specific AI comes into the picture, providing an impactful solution to these obstacles. The generic AI systems are designed to work across all the industries. On the other hand, domain-specific AI is designed to meet the unique needs of specific fields, be it finance, manufacturing, healthcare, or retail. A domain-specific AI focuses on the complexities and nuances of a specific industry to identify and realize the full potential of data, thus enabling various businesses drive success by making timely decisions with greater insights. Domain-specific AI is important and hard to ignore in a world where speed and precision are everything.
How domain-specific AI work?
The working of domain-specific AI goes beyond generic algorithms by understanding the complexities and intricacies of a specific industry. Please have a look at how the domain specific AI works in a more specialized manner:
1. Industry-centric data ingestion: Domain-specific AI pulls in more relevant, industry-specific datasets instead of depending on generic data sources. For instance, in the legal field, employing domain-specific AI might analyze past case rulings, while in the e-commerce industry, it might emphasize on consumer behaviour patterns and purchase histories.
2. Contextual understanding: The domain-specific AI is trained not just on the data but on the context surrounding it. In healthcare, for instance, the AI doesn’t just process medical data but understands the clinical nature of symptoms, patient history, and treatments. This helps the AI to devise solutions that align closely with the practical world applications.
3. Adapted algorithms and workflows: Domain-specific algorithms do not fall under one-size-fits-all models category. Instead, it uses algorithms that are fine-tuned to the specific challenges of the domain. In financial industry, it might use predictive models to assess market risks; whereas in manufacturing world, it might concentrate on optimizing supply chain efficiency.
4. Continuous feedback loop: Domain-driven AI thrives on continuous feedback. The AI interacts with real-world applications and gathers insights from actual usage, thus refining its recommendations to remain consistent with industry shifts, regulatory changes, or emerging patterns.
5. Expert collaborations: These domain-specific AI systems are frequently built with association from domain experts. These experts inject knowledge into the development process. This makes the system intelligence-driven apart from data-driven, ensuring the decisions reflect both AI insights as well as human expertise.
The benefits of domain-specific AI
1. Higher accuracy and reliability: One of the standout benefits of domain-specific AI is its ability to achieve tremendous accuracy. For instance, Evaluserve has come up with Spreadsmart solution that operates at a 99% accuracy rate, which is significantly higher than traditional and manual methods. This remarkable precision is because of AI models that are being trained on industry-specific data and allow for insights that are meaningful and executable.
2. Faster time to impact: Domain-specific AI speeds up the implementation times. Various companies have developed automation platforms that deploy customer analytics models in shorter timeframes such as weeks instead of months required to build them from scratch. This faster deployment means organizations can start viewing the benefits of AI quickly, be it the improvement in decision-making process, optimizing workflows or increasing operational efficiency.
3. Access to pre-trained data from multiple sources: Domain-specific AI excels by utilizing pre-trained models, unlike generic AI systems that often struggle with insufficient data information. Organizations are working with multiple clients within the same industry or functional domain, This allows them to compile datasets from diverse sources. This broader data pool strengthens the models and makes them more resilient, even when the individual clients lack sufficient data.
4. Unconstrained by training data limitations: Without the typical constraints related to limited or inconsistent training data, domain-specific AI is built to work efficiently and accurately. This removes one of the primary barriers many businesses face when adopting AI, ensuring that the models function optimally from day one.
5. Cost-effective and scalable solutions: Using general AI to solve domain-specific problems can be costly and inefficient, especially when businesses face challenges such as frequent changes in data or insufficient training data. Domain-specific AI is a cost-effective, scalable solution that offers more reliable, impactful results. Whether addressing strategic decisions or scaling across an enterprise, domain-specific AI ensures businesses can achieve faster, more accurate outcomes, all while minimizing costs.
Limitations of a domain-specific AI
While domain-specific AI offers significant advantages, it also comes with certain limitations. One challenge is its limited adaptability. These models are finely tuned to a particular industry or function, which means they may struggle to perform well outside of that context. This can hinder scalability aspect if a business intends to expand its AI use across multiple domains.
Another limitation could be the dependency on high-quality data. Although domain-specific AI benefits from pre-trained models, the quality of the data used for training is critical. In industries where the data is sparse or inconsistent, the AI’s performance may be compromised.
Additionally, implementation costs can be higher, as customizing AI models to fit specific needs often requires significant upfront investment in data collection, model development, and industry expertise. Lastly, while the AI may perform excellently in one sector, maintaining relevance requires continuous updates to adapt to evolving industry trends and regulations.
Conclusion
Domain-specific AI offers a dynamic tool for organizations to meet their industry-specific needs, speedily, accurately and even more efficiently. Tailored models and industry-specific data make it possible for organizations to expect reliable results, streamline processes, and optimally extract returns on their investments. As industries continue to evolve, the organizations holding the capability to deploy finely tuned Artificial Intelligence solutions to specific needs will enjoy competitive edge and remain agile and ahead of the pack. In the end, domain-specific AI caters to the needs of its users and produces smarter decisions for leaders, ensuring sustained success and growth of the business.
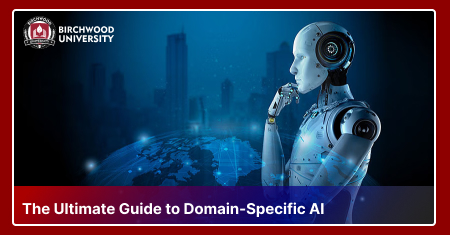