How to Use AI and Real-Time Machine Learning to Make Strategic Business Decisions
October 24, 2024 2024-10-25 8:54How to Use AI and Real-Time Machine Learning to Make Strategic Business Decisions
As we exist in a digital realm where data reigns supreme, what methods can organizations employ to mobilize the power of artificial intelligence (AI) and real-time machine learning to make strategic business decisions? As businesses face an ever-increasing volume of data and dynamic market developments, the ability to make informed, agile decisions becomes paramount. This article explores the integration of AI and machine learning into business strategies, highlighting their transformative impact on decision-making processes.
Role of AI and real-time machine learning in business
Artificial intelligence refers to the simulation of human intelligence in machines programmed to think and learn. It encompasses various technologies, including natural language processing, computer vision, and machine learning. For businesses, AI can automate processes, enhance customer experiences, and provide deeper insights through data analysis.
Real-time machine learning involves the continuous analysis of data as it is generated. This helps businesses to adapt their strategies instantaneously. Unlike traditional machine learning, which often relies on historical data, real-time learning processes incoming data streams, identifying patterns and making predictions without delay. This capability is particularly valuable in dynamic environments where swift decision-making is essential.
Strategic advantage of AI and real-time machine learning
1. Augmenting strategic choices with data
According to McKinsey, organizations that prioritize data-driven strategies are 23 times more likely to acquire new customers. Moreover, they are six times more likely to retain existing customers, and 19 times more likely to achieve profitability. Businesses can capitalize on this data to influence smart choices and improve the customer experience. This leads to satisfied customers who return consistently, promoting continuous growth and enduring success. AI and machine learning strengthen this data-driven approach by empowering businesses to analyze vast amounts of data quickly and efficiently. These actionable propositions assist organizations to make appropriate decisions that are in sync with market demands.
2. Predictive analytics for informed forecasting
Predictive modeling, powered by machine learning algorithms, enables businesses to anticipate future trends and behaviours. For instance, retail companies can analyze customer purchasing patterns to forecast demand, optimize inventory levels, and customize marketing plans. Predictive analytics can help organizations to stay ahead of the competition and allocate resources more effectively.
3. Personalized customer experiences
Adapting services and products to individual preferences is essential for keeping customers loyal. AI can analyze customer behavior and preferences to create personalized experiences, from targeted marketing campaigns to curated product recommendations. According to a study by Epsilon, 80% of consumers are more likely to make a purchase when brands offer personalized experiences. This level of customization, driven by real-time machine learning, deepens customer affiliations and boosts sales.
Implementing AI and machine learning in business strategies
1. Identifying important business areas for AI integration
- Customer service: AI-powered chatbots can provide instant responses to customer inquiries, improving satisfaction and reducing operational costs.
- Supply chain management: Machine learning algorithms can optimize supply chain processes by predicting demand fluctuations and identifying potential disruptions.
- Marketing and sales: AI can analyze customer data to refine targeting strategies, improving the effectiveness of marketing campaigns.
- Risk management: Organizations can utilize AI to assess risks in real-time, enabling proactive decision-making and minimizing potential losses.
2. Building a resilient data infrastructure
Businesses must invest in an adaptive data architecture for AI and machine learning to deliver meaningful insights.
- Data collection: Implementing systems to collect relevant data from various sources, such as customer interactions, sales transactions, and social media engagement.
- Data storage: Utilizing cloud-based storage solutions to manage large volumes of data securely and accessibly.
- Data governance: Establishing protocols to ensure data quality, security, and compliance with regulations.
3. Selecting the right tools and technologies
Choosing which AI and machine learning tools is vital for successful implementation. Organizations should consider:
- Machine learning platforms: Solutions such as TensorFlow, Microsoft Azure ML, Amazon SageMaker offer immense capabilities for building and deploying machine learning models.
- Data visualization tools: Platforms such as Tableau and Power BI help businesses visualize data trends and insights, facilitating better decision-making.
- AI-based analytics: Tools such as Google Analytics and IBM Watson can provide advanced analytical capabilities to derive tangible recommendations.
4. Training and upskilling employees
The successful integration of AI and real-time ML requires a skilled workforce. Organizations should invest in training and upskilling employees to ensure they comprehend how to effectively utilize these technologies. This may include:
- Workshops and seminars: Hosting training sessions to familiarize staff with AI tools, machine learning techniques and methodologies.
- Online courses: Encouraging employees to pursue online courses in data science and machine learning to enhance their expertise.
- Cross-department collaboration: Developing teamwork between IT and business teams to ensure that AI initiatives align with organizational goals.
Prospects of AI and real-time ML in strategic business decisions
Future developments in this area may include:
- Enhanced natural language processing: Improved language models will enable businesses to analyze customer sentiments and feedback more accurately.
- Autonomous decision-making systems: Organizations may develop AI systems capable of making instant decisions without human intervention, further streamlining operations.
- Greater integration with IoT: The convergence of AI and the Internet of Things (IoT) will allow businesses to gather real-time data from connected devices, refining their decision-making proficiency.
Barriers to AI and real-time ML integration
Implementing AI and real time machine learning in business strategies faces several barriers:
- Data availability: Access to high-quality, relevant data is critical. Organizations often struggle with data silos, where information is trapped within departments, hindering real-time analysis.
- Data quality: Inconsistent, incomplete, or outdated data can lead to inaccurate predictions. Ensuring data integrity is essential for effective real-time machine learning.
- Infrastructure challenges: Businesses may lack the necessary technological infrastructure to support real-time data processing and analytics, requiring significant investment in hardware and software.
- Integration issues: Seamlessly integrating AI and real-time ML systems with existing business processes and platforms can be complex and resource-intensive.
- Skill gaps: There is often a shortage of professionals skilled in AI and real-time ML, making it difficult for organizations to fully leverage these technologies.
- Regulatory compliance: Adhering to data privacy regulations adds further complications, as companies must ensure that real-time data usage complies with legal standards.
Conclusion
In conclusion, integrating AI and real-time machine learning has become essential for organizations that want to remain competitive. The future of business depends on the quick and strategic choices that these technologies enable. Adopting these innovations may be vital for achieving success in a constantly changing business environment.
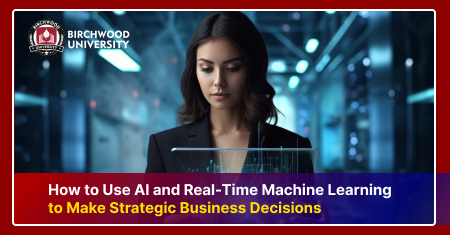