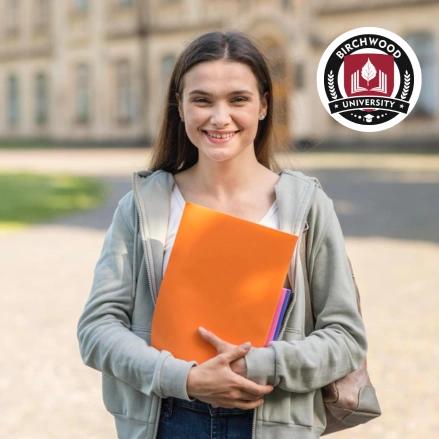
About The University
Welcome to Birchwood University!
Your educational journey begins here at Birchwood University, where you will receive the quality education needed for the fast-paced changing world.
- Birchwood University helps you to develop the skills and knowledge necessary to transform the workforce.
- Our fully accelerated online degree programs that offers flexibility to earn your degree while working.
- Our dedicated faculty and staff will focus on your success so that you can achieve your full potential.
Get Supportinfo@birchwoodu.org
Why Choose Us ?
Why Choose Birchwood University?
We want to transform the workspace of the future.
Our Latest
Upcoming Events
Acquire the latest update with innovative occurrences and figure out the supreme part of our current activities.
No Upcoming Events.
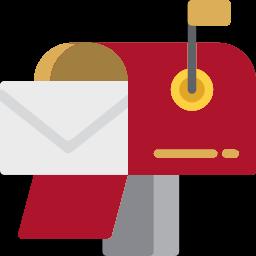
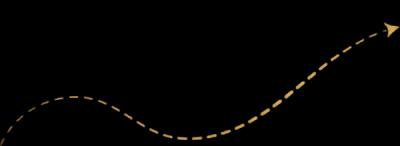
Latest News
Latest blogs & articles
Check out all the latest blogs & articles about Birchwood University.